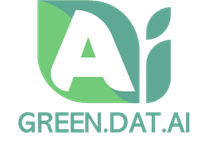
Green.Dat.AI – Energy-efficient AI-ready Data Spaces
GREEN.DAT.AI aims to channel the potential of AI towards the goals of the European Green Deal, by developing novel Energy-Efficient Large-Scale Data Analytics Services, ready-to-use in industrial AI-based systems, while reducing the environmental impact of data management processes. GREEN.DAT.AI will demonstrate the efficiencies of the new analytics services in four industries (Smart Energy, Smart Agriculture/Agri-food, Smart Mobility, Smart Banking) and six different application scenarios, leveraging the use of European Data Spaces. The ambition is to exploit mature (TRL5 or higher) solutions already developed in recent H2020 projects and deliver an efficient, massively distributed, open-source, green, AI/FL – ready platform, and a validated go-to-market TRL7/8 Toolbox for AI-ready Data Spaces. The services will cover AI-enabled data enrichment, Incentive mechanisms for Data Sharing, Synthetic Data Generation, Large-scale learning at the Edge/Fog, Federated & Auto ML at the edge/fog, Explainable AI/Feature Learning with Privacy Preservation, Federated & Automatic Transfer Learning, Adaptive FL for Digital Twin Applications, Automated IoT event-based change detection/ forecasting. The GREEN.DAT.AI Consortium consists of a multidisciplinary group of 17 partners from 10 different countries (and one associated party), well balanced in terms of expertise.
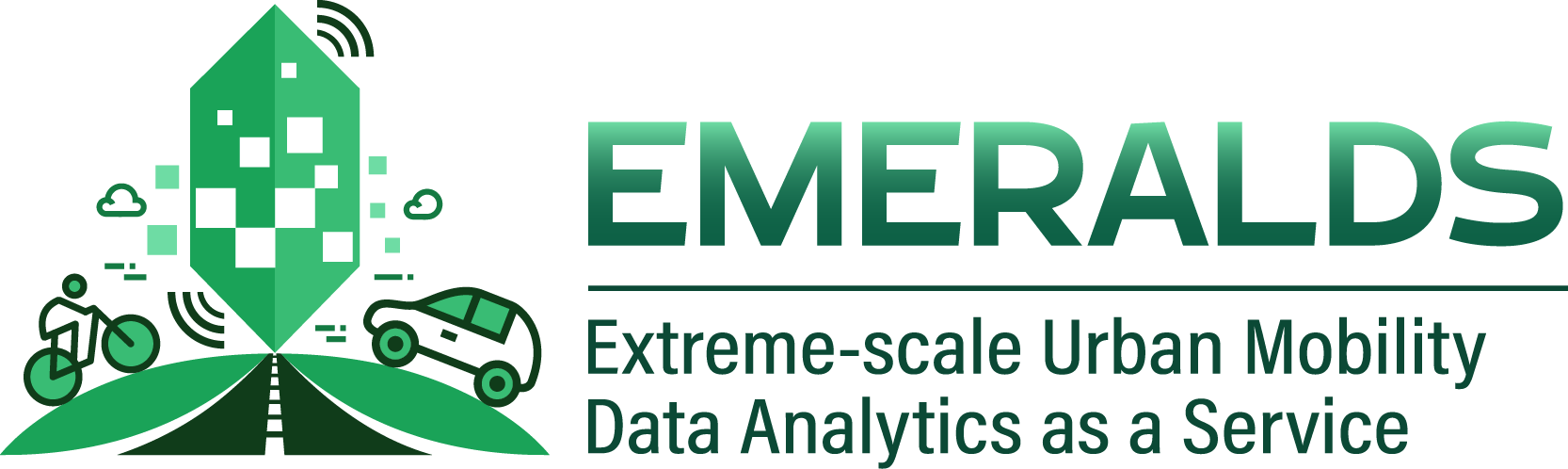
EMERALDS – Extreme-scale Urban Mobility Data Analytics as a Service
EMERALDS’s vision is to design, develop and create an urban data-oriented Mobility Analytics as a Service (MAaaS) toolset, consisting of the so-called ‘emeralds’ services, compiled in a proof-of-concept prototype, capable of exploiting the untapped potential of extreme urban mobility data. The toolset will enable the stakeholders of the urban mobility ecosystem to collect and manage ubiquitous spatio- temporal data of high-volume, high-velocity and of high-variety, analyse them both in online and offline settings, import them to real-time responsive AI/ML algorithms and visualize results in interactive dashboards, whilst implementing privacy preservation techniques at all data modalities and at all levels of its architecture. The toolset will offer advanced capabilities in data mining (searching and processing) of large amounts and varieties of urban mobility data and its efficiency will be assessed, validated and demonstrated in three TRL5 pilot use cases (by following a co-development approach with mobility and city stakeholders to improve decision making in urban smart city environments), and deployed/showcased in two early adopters’ data-driven TRL6 applications (by integrating the new services to existing systems to improve commercial offerings).
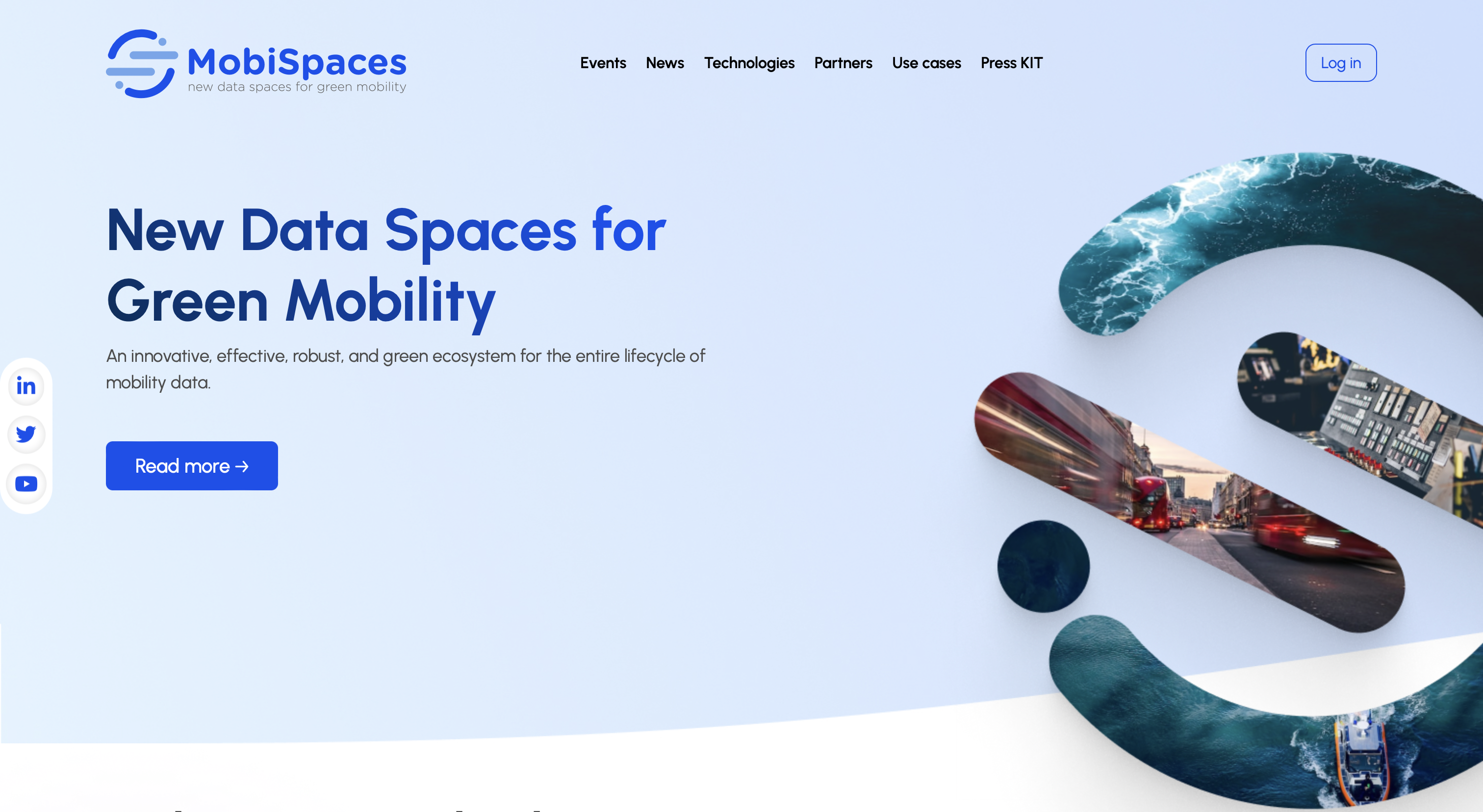
MobiSpaces, New Data Spaces for Green Mobility
Mobility in the urban and maritime domains hugely impacts the global economy, generating data at high rates from an increasing number of moving objects. Management of the complete lifecycle of such data implies that trustworthy and privacy-preserving infrastructures need to be put in place, so that reliable and secure data operations can be provided. Meanwhile, the mobility data exploitation still has a wide potential due to the emerging applications and the environmental footprint caused by mobility.
From September 2022 to August 2025, the brand new Horizon Europe project MobiSpaces will be developing effective data governance solutions to exploit the huge data volumes produced in secure and trustworthy digital infrastructures to enable data sharing, reuse and interoperability using standardised protocols across different organisations and stakeholders.
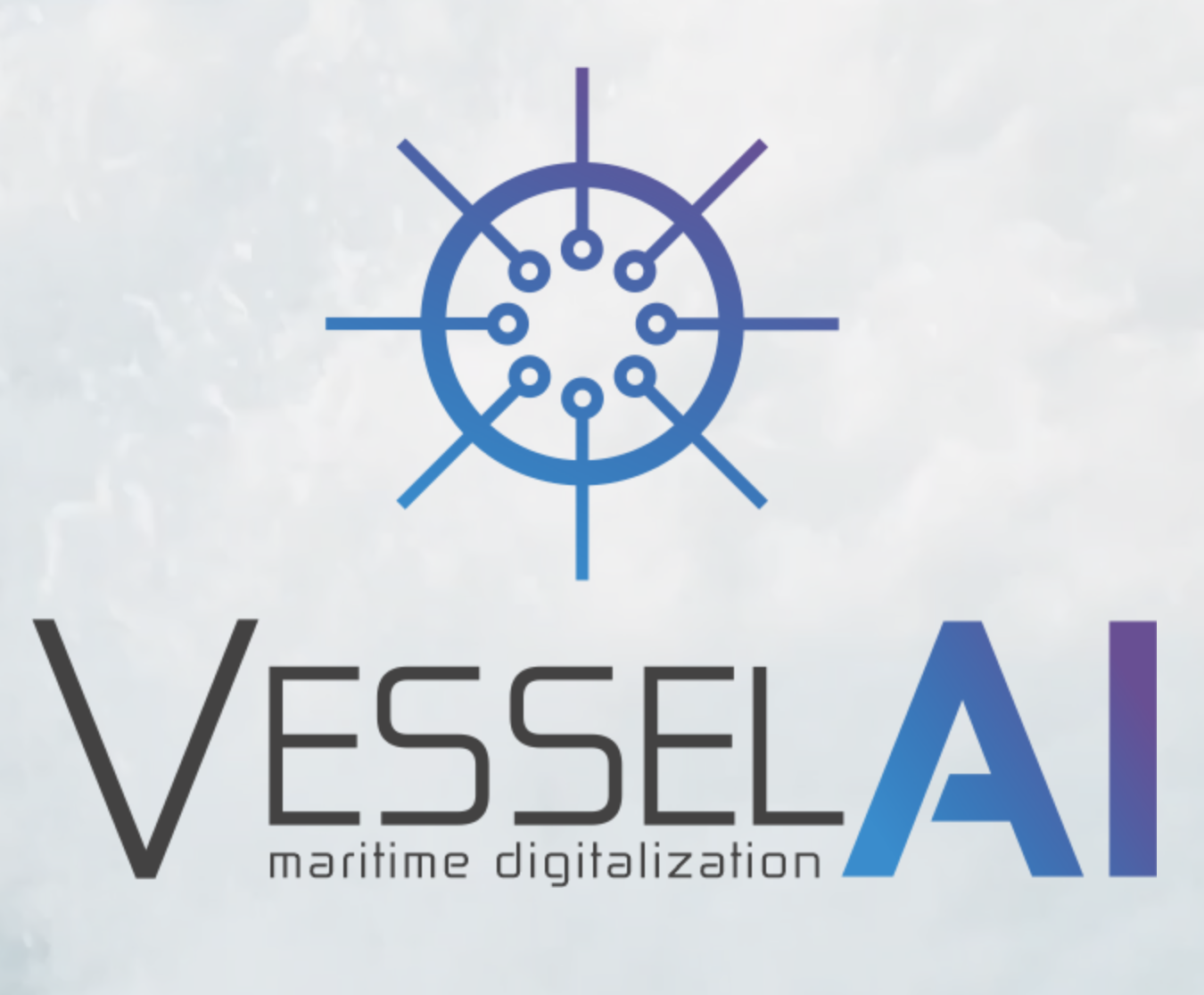
VesselAI – Enabling Maritime Digitalization by Extreme-scale Analytics, AI and Digital Twins
VesselAI aims at realising a holistic, beyond the state-of-the-art AI-empowered framework for decision-support models, data analytics and visualisations to build digital twins and maritime applications for a diverse set of cases with high impact, including simulating and predicting vessel behaviour and manoeuvring (including the human factor), ship energy design optimisation, autonomous shipping and fleet intelligence.
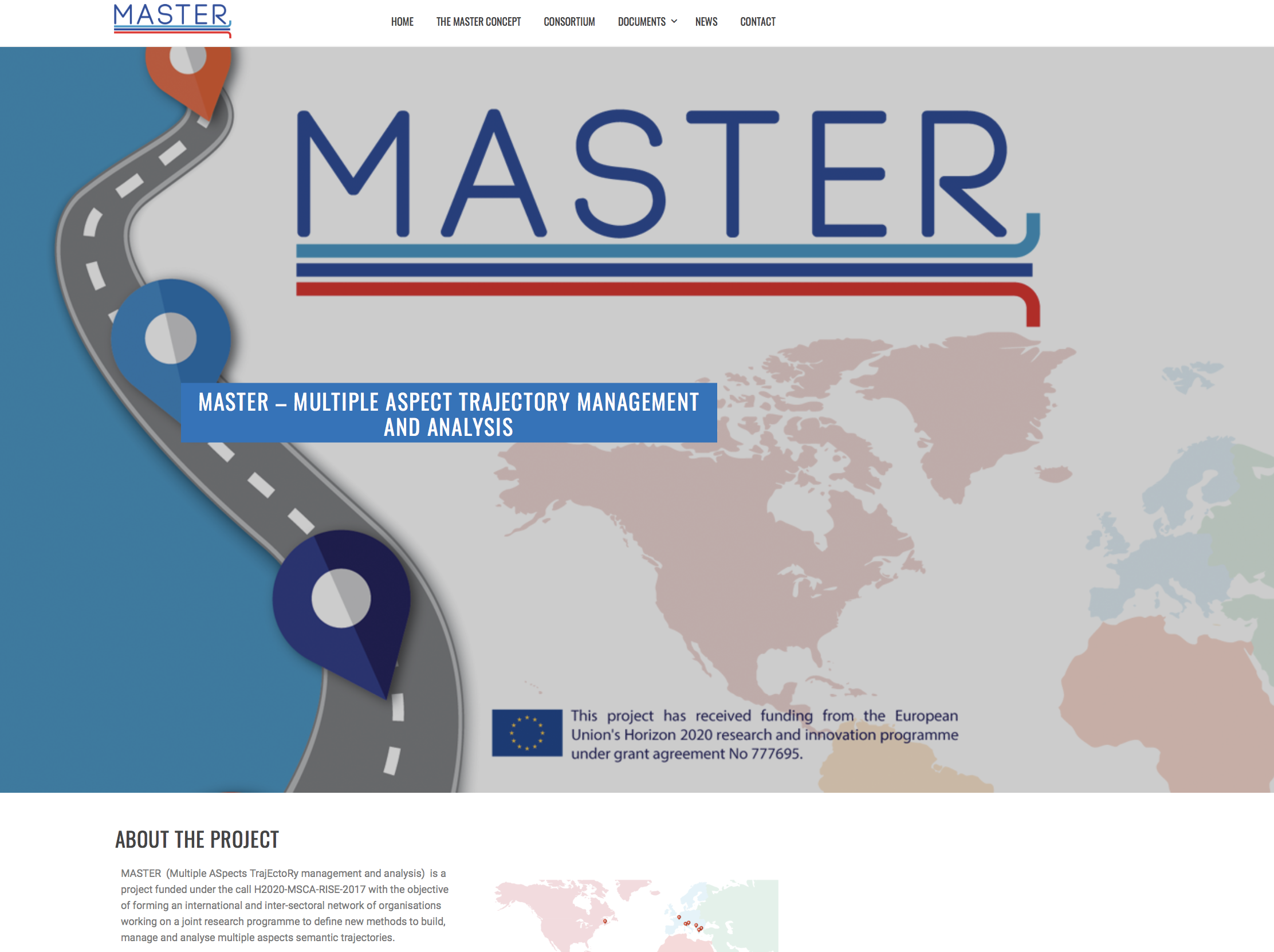
MASTER – Multiple ASpects TrajEctoRy management and analysis
MASTER (Multiple ASpects TrajEctoRy management and analysis) is a project funded under the call H2020-MSCA-RISE-2017 with the objective of forming an international and inter-sectoral network of organisations working on a joint research programme to define new methods to build, manage and analyse multiple aspects semantic trajectories.