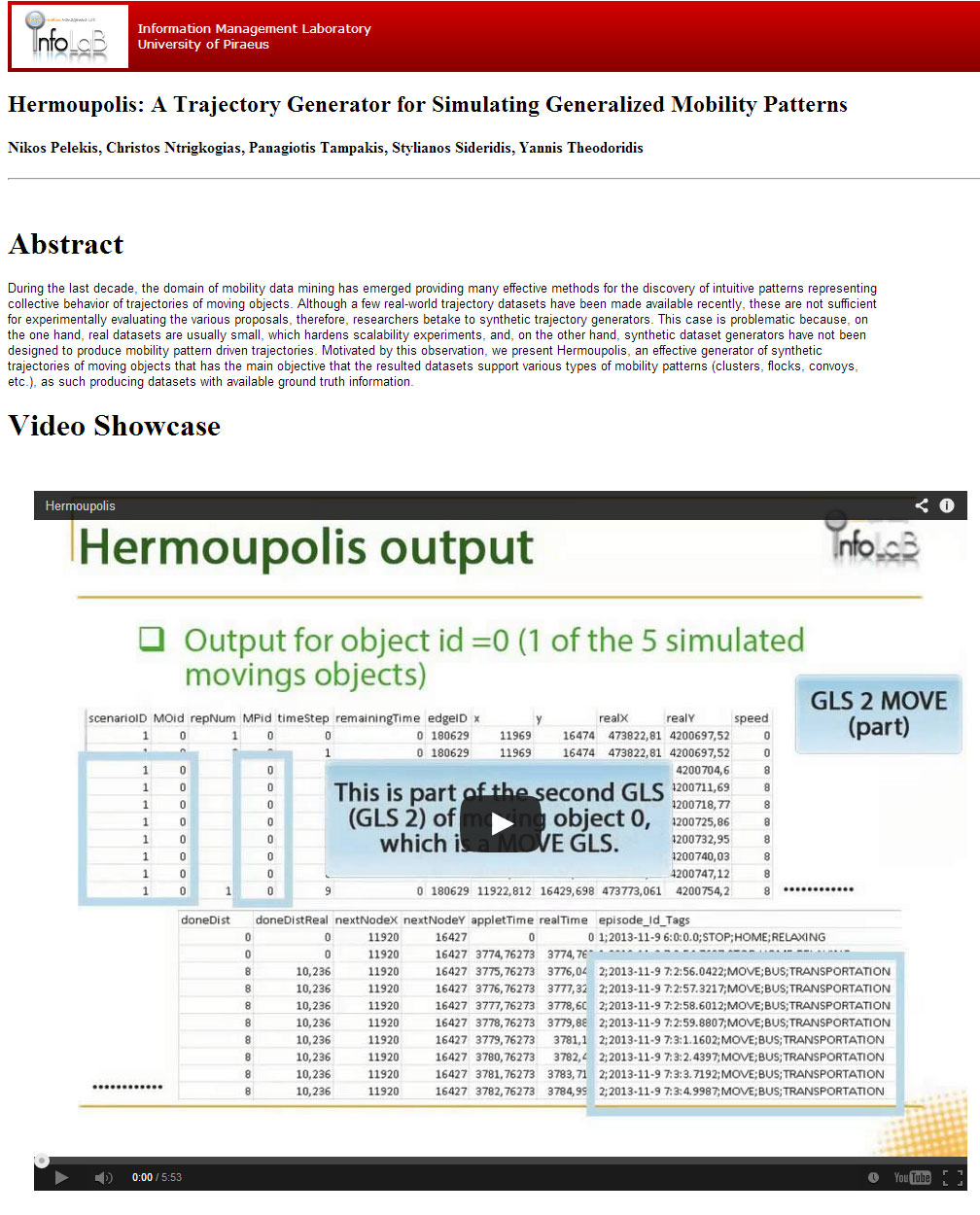
During the last decade, the domain of mobility data mining has emerged providing many effective methods for the discovery of intuitive patterns representing collective behavior of trajectories of moving objects. Although a few real-world trajectory datasets have been made available recently, these are not sufficient for experimentally evaluating the various proposals, therefore, researchers betake to synthetic trajectory generators. This case is problematic because, on the one hand, real datasets are usually small, which hardens scalability experiments, and, on the other hand, synthetic dataset generators have not been designed to produce mobility pattern driven trajectories. Motivated by this observation, we present Hermoupolis, an effective generator of synthetic trajectories of moving objects that has the main objective that the resulted datasets support various types of mobility patterns (clusters, flocks, convoys, etc.), as such producing datasets with available ground truth information.